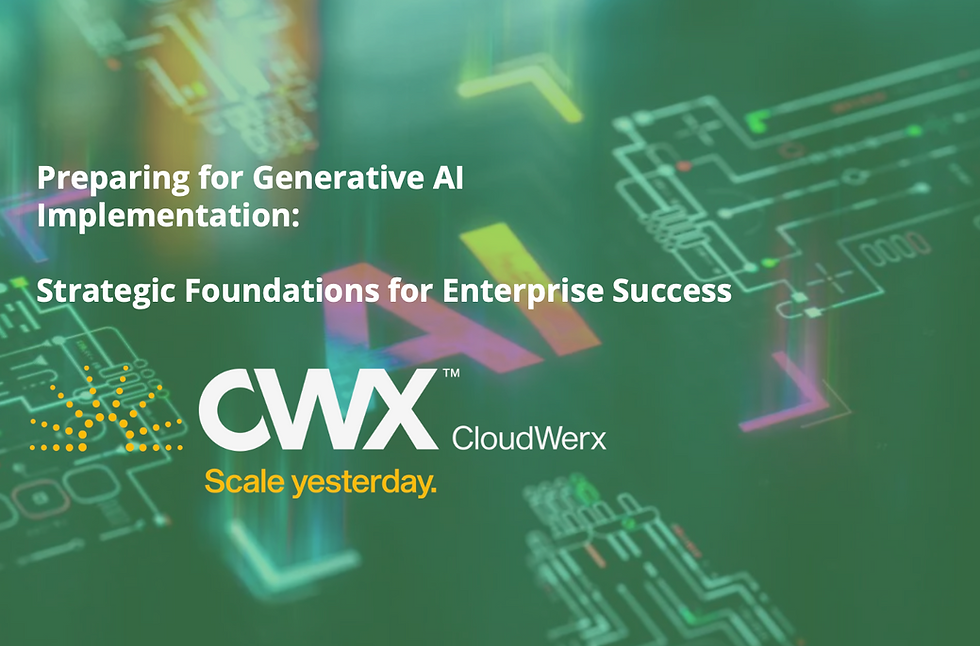
As generative AI (GenAI) rapidly transforms industries, organizations are racing to integrate these powerful tools into their operations. However, successful implementation requires more than selecting the right software—it demands careful preparation to ensure long-term value, scalability, and ethical alignment.
Key steps to consider before introducing GenAI include evaluating data readiness, ensuring secure platforms, and establishing ethical guidelines for responsible AI usage. Here’s a closer look at these foundational elements and why they are critical for enterprises aiming to maintain a competitive edge.
Data Readiness for AI (DRAI): Laying the Groundwork
Generative AI systems thrive on high-quality data. However, many organizations overlook the importance of preparing their datasets for seamless AI integration. The concept of Data Readiness for AI Ingestion (DRAI) involves assessing whether the organization’s data is in an AI-ready format. Data readiness is crucial for enterprises implementing AI systems, as it directly impacts the accuracy, reliability, and effectiveness of AI models. Poor quality data inevitably leads to inaccurate and unreliable results, embodying the "garbage in, garbage out" principle. A Scale AI survey revealed that many organizations face significant challenges with data readiness in their machine-learning projects, with data quality emerging as the biggest hurdle.
With AI technologies projected to contribute up to $15.7 trillion to the global economy by 2030, investing in data readiness has become a strategic imperative rather than just a technical necessity[5]. Organizations that prioritize data readiness can better leverage AI technologies for growth and innovation, while those that neglect it risk project failures and compromised decision-making. Gartner estimates that the average enterprise loses $12.9 million annually due to poor-quality data, highlighting the significant financial impact of inadequate data preparation.
AI-ready data must be:
Clean and well-structured: Datasets should be free from duplicates, errors, or inconsistencies that could distort AI outputs.
Accessible: Data silos often impede AI projects. Unified data repositories or integration layers can solve this problem.
Relevant: Data must align with the organization’s specific GenAI use cases, whether generating customer insights, automating content creation, or enhancing decision-making.
In a case study by MIT Technology Review, a major financial services company achieved a 20% productivity boost in customer support by first standardizing and annotating its datasets before deploying a GenAI tool. This step prevented errors and increased system reliability.
Enterprises should audit their data pipelines and work with data scientists to identify gaps before AI deployment. Investing in data readiness will significantly improve the quality and reliability of AI outputs.
Building GenAI Solutions on Secure Platforms
Security breaches and data leaks are growing risks as AI solutions expand access to sensitive data. Enterprises must ensure their GenAI tools are built on secure and compliant platforms. As enterprises rapidly embrace AI technologies, several critical security aspects have emerged as paramount in 2023-2024. The integration of AI has fundamentally altered enterprise infrastructure, creating complex security challenges that require immediate attention.
Key considerations for secure AI implementation include:
Data Protection: Organizations must implement robust security measures to protect AI models and datasets from unauthorized access, manipulation, or theft. This includes encryption, authentication protocols, and comprehensive audit logs.
Infrastructure Security: The AI infrastructure market, projected to reach $96 billion by 2027, requires secure-by-design approaches and continuous monitoring. Organizations must implement resilient security measures across their entire AI ecosystem.
Compliance and Governance: With AI adoption rising 250% from 2017 to 2022, enterprises must establish robust policies for evaluating, approving, and monitoring AI applications while ensuring compliance with regulations like GDPR and HIPAA.
Threat Detection: AI-powered security systems are increasingly vital for identifying and responding to sophisticated cyber threats in real-time, particularly as cybercriminals leverage AI for more advanced attacks.
Establishing Ethical Guidelines and Responsible AI Practices
AI’s immense power comes with ethical responsibilities. Enterprises deploying GenAI tools must prioritize fairness, transparency, and accountability. Establishing ethical guidelines ensures that AI systems are used responsibly and without unintended harm.
Key components of responsible AI include:
Bias Mitigation: Algorithms trained on biased data can perpetuate inequalities. Organizations should audit datasets and implement fairness metrics during development.
Transparency: Clear documentation of how the AI system operates builds trust among users and stakeholders.
Accountability: Define who is responsible for the outcomes of AI-powered decisions, particularly in high-stakes industries like healthcare or finance.
Google has established a comprehensive AI ethics framework that emphasizes regular audits and a commitment to minimizing bias in its AI systems. This proactive approach includes the development of internal algorithmic auditing practices to ensure accountability and fairness.
Additionally, Google has outlined its AI Principles, which guide the responsible development and use of AI technologies, focusing on fairness, transparency, and accountability. These initiatives have reinforced Google's reputation as a leader in responsible AI development.
Enterprises should also form cross-functional committees—including legal, HR, and tech leaders—to oversee AI ethics and align GenAI initiatives with organizational values.
Continuous Evolution of Strategic Implementations
As AI technologies evolve, so too will the strategies required for their successful deployment. Regularly revisiting and refining these foundational elements—data readiness, secure platforms, and ethical guidelines—will help enterprises adapt to new challenges and opportunities.
Emerging trends to monitor include:
AI Governance Tools: These platforms offer automated auditing, explainability, and bias detection for AI systems, simplifying compliance with ethical guidelines. Source: Gartner
Zero Trust Architecture for AI Systems: This approach minimizes security risks by continuously verifying access permissions within AI workflows.
Evolving Regulatory Frameworks: Governments worldwide are introducing AI-specific regulations. Staying ahead of compliance will minimize disruptions.
Conclusion: Positioning for GenAI Success
Generative AI offers transformative potential, but its benefits are only realized through deliberate preparation and ongoing management. By focusing on data readiness, secure platforms, and ethical guidelines, enterprises can lay the groundwork for sustainable AI innovation.
These steps not only enhance the reliability and security of AI systems but also ensure alignment with broader organizational goals, including customer satisfaction and long-term competitiveness. CEOs who champion these initiatives will lead their organizations confidently into the AI-driven future.
Comments